A data-driven framework to evaluate design changes in MOOCs
This research aims to develop a data-driven framework to evaluate design changes in MOOCs. We explore a change from multiple angles-process, proficiency, and perception- and apply various analytical methods-temporal, causal and predictive to map out the outcome of instruction along multiple dimensions of learning.
Contribution:
We develop a data-driven framework to evaluate design changes in MOOCs and demonstrate the application of this framework by evaluating course pacing on a repeated run of a supply chain MOOC by MITx. The impact of pacing on students’ outcome was not uniform with some experiencing no change while others encountering a steep fall. The most striking difference was seen in the longitudinal trajectory, with instructor-paced students mostly taking the same pathway and self-paced students pursuing their own individually paced pathways. We showed that these trajectories are correlated with student grade.
Research Publications
- Closing the loop in Learning Analytics – Operationalizing Predictive Models in MOOC Platforms. MIT Master Thesis. SDM Program. August 2019.
Research Team
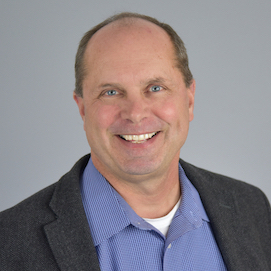
Dr. Chris Caplice
Senior Research Scientist
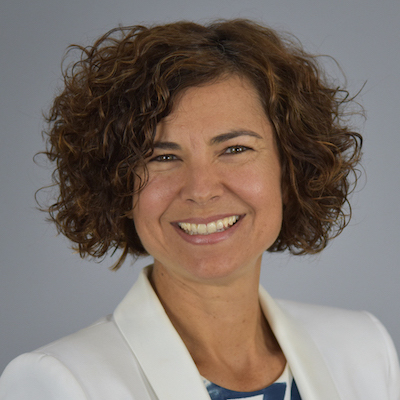
Dr. Eva Ponce
Director
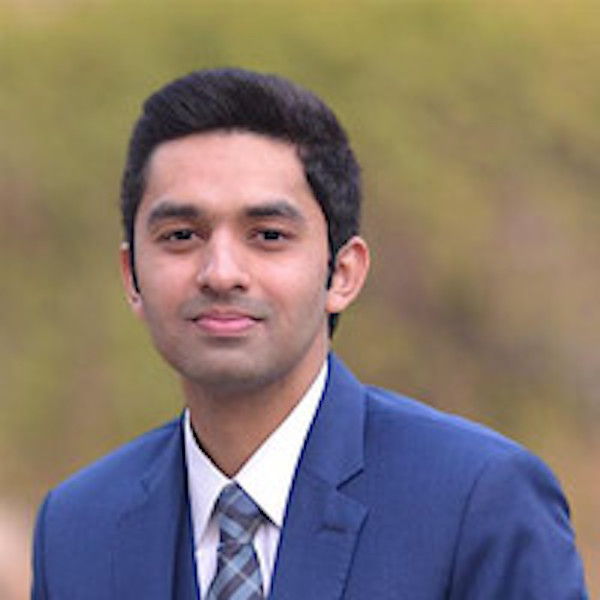
Ahmed Bilal
Research Assistant