Converting zombies into learners
The project aims at increasing learners’ engagement in massive, open, and online education in Supply Chain Management by applying learning analytics.
The research questions:
- How can we predict which verified learners will drop out by analyzing their course activity and performance in MOOCs?
- What actions are effective in reducing the dropout rate?
Main Contributions:
- Develop a predictive model based on machine learning algorithms to predict learners at risk of dropout. Our models use click-stream data as a proxy for learners’ activity in the course. We followed a chronological approach, training our models in older course runs and testing them in more recent course runs. We used two very well-known machine learning algorithms: logistic regression and random forests. These algorithms are known for their robustness, ability to identify most relevant predictors, and high predictive power. We tested these algorithms based on their recall values. Our model was able to predict four out of five actual dropouts in the courses that were part of our sample.
- Develop a framework to identify relevant dropout factors when designing and classifying interventions in massive open and online courses and programs.
- Design and implement four experimental studies (interventions) to reduce the dropout rate in massive and open online courses through student interventions and course design interventions
Key Findings:
Our predictive model included 80 predictors. The most relevant ones to predict learners at risk of dropout a massive and open online course are grades and time spent in the course. The use of recall metrics and a chronological approach to build predictive models would lead to more useful models for real settings. Our model was able to predict four out of five actual dropouts in the courses that were part of our sample. Our experiments showed that course design interventions are more effective than student interventions to reduce the dropout rate.
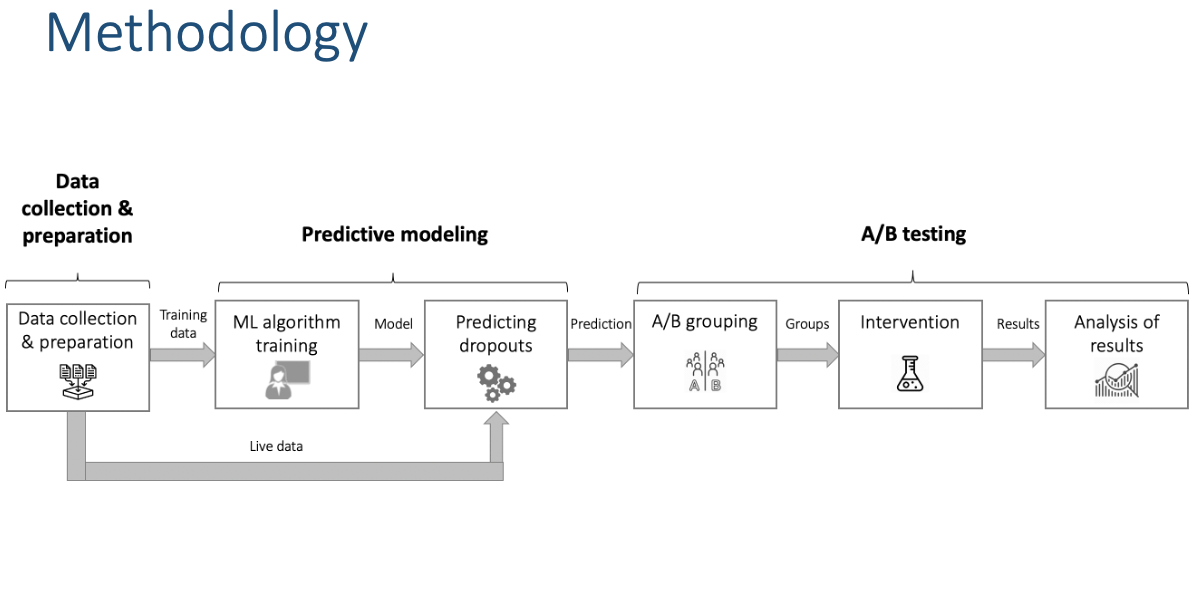
Research Publications
- Borrella, I.; Caballero-Caballero, S., Ponce-Cueto, E. (2019). Predict and Intervene: Addressing the Dropout Problem in a MOOC-based Program. Learning @Scale 2019, Chicago, June.
- Borrella, I.; Caballero-Caballero, S., Ponce-Cueto, E. (In-progress). Analyzing the effectiveness of interventions to increase learners’ engagement in MOOC-based programs. Computers & Education. Elsevier Journal.
- Ponce, E. (2019). Omnichannel Education: Are You Ready for the Challenge? Supply Chain Management Review, December 10, 2019.
Conferences/Invited talks:
- Ponce, E., Srinath, S., Allegue, L. Integrating Community Teaching in MOOCs. L@Scale / eMOOCs, 22-24 June, 2021, Online Conference, pp. 95- 109 – https://publishup.uni-potsdam.de/opus4-ubp/frontdoor/deliver/index/docId/51030/file/emoocs2021.pdf
- Ponce, E. (2019). DSI (Decision Sciences Institute Annual Meeting) 50th Annual Conference – Innovative Teaching Track – The Omni-channel of course delivery – conventional, online, MOOCs, hybrid/blended, and the premium each can offer – New Orleans, LA. November 23-25.
- Ponce-Cueto, E., Caplice, C. (2019). MicroMasters are Different: Lessons from the first MicroMasters Program. eMOOCS conference. Naples. May 20-22, 2019.
- Golara, S.; Ponce, E. (2018) Conversion Rate From Audit to Paid Membership in Massive Open Online Courses. 49th Annual Meeting of the Decision Sciences Institute. November, 17-19, 2018.
- Ponce, E. (2019). Online Learning Innovations and Interventions. XTALKS: DIGITAL DISCOURSES, MIT, Cambridge, MA, April 30.
- Ponce, E. (2019). Online Learning and Blended Education in SCM. Teaching/pedagogy track 2019. POMS (Production and Operations Management Society) 27th Annual Conference, Washington D.C., May 2-6
Research Team
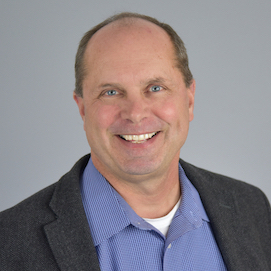
Dr. Chris Caplice
Senior Research Scientist
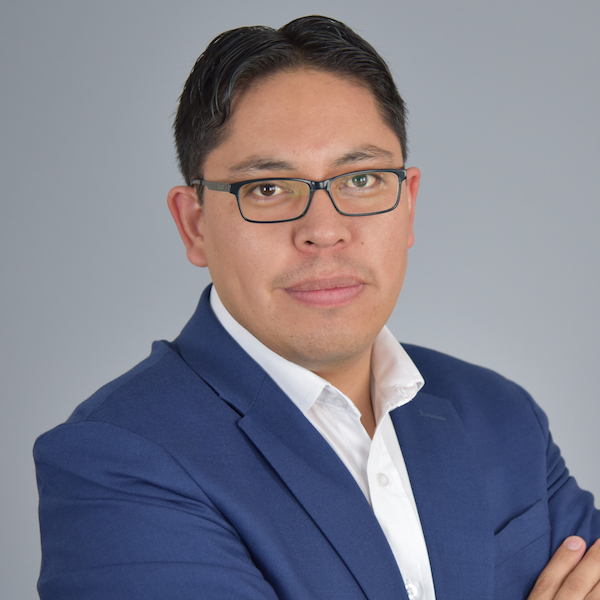
Dr. Sergio Caballero
Research Scientist
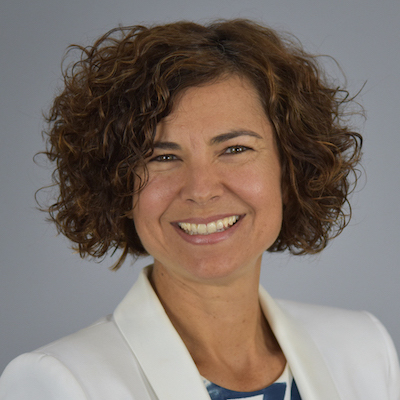
Dr. Eva Ponce
Director
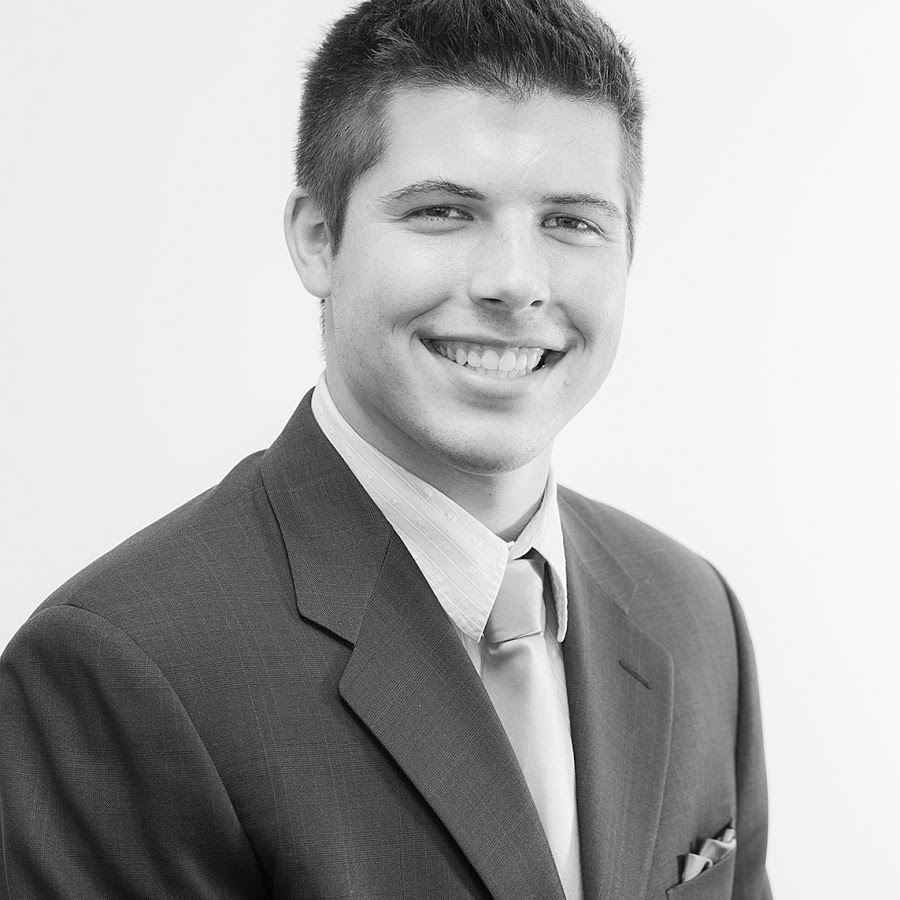
Connor Makowski
Digital Learning Lead
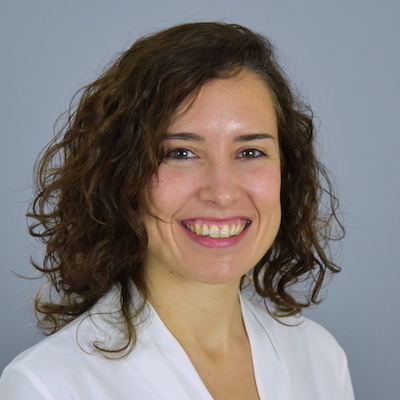
Dr. Inma Borrella
Research Scientist